Written by: Varvara Soloveva
Generative AI is transforming the digital product development lifecycle (PDLC) at an unprecedented pace, reshaping productivity, quality, and processes across industries. While the technology sector has been the primary beneficiary, other sectors that are still undergoing digital transformation, such as banking, have the potential to realize substantial gains as well. GenAI applications could reduce the time needed to ideate, code, test, and launch new features, enabling faster and more impactful product delivery. For instance, GenAI tools for developers, such as GitHub Copilot, are already gaining significant traction for their ability to streamline coding tasks. Moving forward, AI tools will be increasingly integrated across the entire product lifecycle—from customer / market research, ideation, coding and testing to optimization and maintenance. Yet, buying licenses for tools alone is not sufficient; organizations must also focus on driving effective usage and developing metrics to measure success in order to fully capture the value of GenAI.
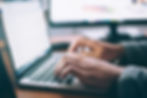
Transforming the Product Development Lifecycle (PDLC)
Generative AI could significantly enhance the PDLC by improving productivity and quality at every stage, from ideation to development and deployment.
In the ideation phase, GenAI could enhance the breadth of ideas for product managers. AI tools can generate comprehensive user stories, tailored with high-fidelity context understanding. By suggesting numerous variations, these suggestions help development teams rapidly validate concepts and align with user needs, resulting in faster realization and validation of new features. GenAI can also enable more inclusive brainstorming sessions by offering suggestions that bring diverse perspectives, helping teams consider possibilities that may have otherwise been overlooked.
During development, GenAI augments developer tasks by handling routine coding and testing activities. Tools like GitHub Copilot, Codium, and Amazon CodeWhisperer assist with code generation, refactoring, and unit tests, enabling developers to focus on higher-level problem-solving and richer design. This reduces development timelines and ensures that code evolves continuously based on updated requirements. Moreover, developers gain the advantage of AI-assisted code reviews that help maintain consistent quality and adherence to best practices, reducing technical debt over time.
GenAI is transforming deployment and maintenance with proactive monitoring and automation capabilities. Integrated into the deployment phase, GenAI can predict issues, analyze customer behavior, and recommend fixes, thus shortening feedback loops and enhancing user satisfaction. By identifying and addressing potential problems early, GenAI not only minimizes downtime but also ensures smoother updates and releases.
Overcoming Adoption Challenges
To fully realize GenAI's benefits, a thoughtful and well-planned approach to change management is essential. A multipronged change management strategy involving centralized communications, peer influence, rewards and recognition, and continuous education is crucial for embedding GenAI into the software development process. By doing so, companies can foster a structured transition to AI-enabled development, ensuring that teams adapt effectively and leverage AI for maximum impact.
Adopting GenAI also requires a cultural shift where teams are encouraged to experiment and iterate, embracing AI-driven insights without the fear of making mistakes. By fostering an environment where learning and adaptation are prioritized, organizations can unlock the full potential of GenAI while empowering their workforce to grow alongside these advanced tools.
Measuring Impact
To understand the value that generative AI delivers, it is important to measure its impact through key performance indicators (KPIs). These metrics can vary across different stages of development:
Discovery and Viability Phases: Product managers can measure the efficiency gains by tracking the time saved from idea generation to issue creation, and by monitoring reductions in rework or increased on-time feature delivery due to well-defined user stories.
Build and Test Phase: Developers can look at metrics like the percentage of boilerplate code generated with AI, which directly correlates to hours saved in manual coding. Test coverage percentages and reductions in refactoring time are other critical indicators of productivity improvements.
Launch & Deploy and Operate & Monitor Phases: For DevOps teams, the time taken from the start of a release to the actual deployment and the number of incorrectly identified issues can serve as good proxies to understand how AI-driven automation is enhancing the software deployment process.
These metrics not only showcase efficiency gains but also emphasize the quality improvements and cost-saving potential of generative AI for digital product development.
Realizing the full potential of GenAI
The rise of generative AI tools in software development is reshaping how digital products are conceived, built, and improved. From fostering creativity during ideation to refining the deployment and monitoring processes, AI is driving significant improvements in productivity, quality, and speed. Companies that effectively integrate these technologies into their product development lifecycle will find themselves better equipped to innovate, adapt, and grow in an increasingly competitive digital landscape.
However, successful adoption hinges on a well-crafted change management strategy that facilitates learning, supports experimentation, and celebrates progress. By strategically implementing generative AI and tracking its impact, companies can unlock new levels of efficiency and quality, paving the way for the next frontier in digital product development.