Written by: Leon Gordon, Executive Contributor
Executive Contributors at Brainz Magazine are handpicked and invited to contribute because of their knowledge and valuable insight within their area of expertise.

Exploring the large quantities of data available to organizations has the potential to introduce a new fact-based era in business innovations such as backing up the innovative ideas to create a data culture backed by solid evidence. However, still, many companies lack a data culture and data is rarely used for organizational decision making. A lack of data, data quality, and users capable of digesting data are amongst the biggest challenges organizations face when trying to establish a data-based culture.
These businesses’ challenges are not technical they are cultural (Tabesh et al., 2019). The approaches are simple enough to use in any organization's decision-making. So, in this article, we are going to discuss the 9 major benefits that can transform the business by establishing a data culture that is considered invincible.
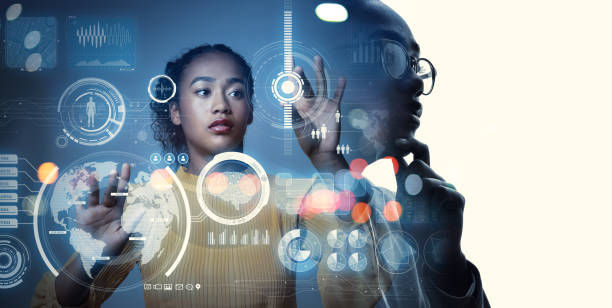
Start Data-Driven culture from top-level
Companies having strong data-driven cultures have top managers that can set expectations in decision-making associated with data. They can start with an example. For example, at one retail bank, the C-suite leaders can shift the evidence through controlled market trials to decide the product launch. At a leading technology firm, the senior executives can spend almost 30 minutes before the start of the meeting reading the whole details of summaries of proposals and the corresponding facts so that they can take valuable action based on the evidence (Heavin et al., 2018). These practices are considered valuable because serious employees can communicate with their senior leaders based on their terms and language. Such practices are considered significant towards substantial shifts in the company norms.
Choose All metrics with care
Leaders can put a powerful impact on the behavior by considering what they have to measure and what metrics they are expecting from the employees to use. For example, a company can earn profit by anticipating its top competitor's price variations. So, it’s a metric for predictive accuracy with time (Sestino et al., 2020). So, a company should continuously have to predict the directions and magnitude of such steps. It will also help to enhance the quality of those predictions that can be slowly improved. For example, a leading telco operator wanted to confirm that its network is providing the best possible user experience to its key customers. But they have only collected the data about network performance. So, how will they know who is receiving it and what is the service quality that customers have experienced? By creating a detailed metric according to customer experience the operator can perform a quantitative analysis of the customer impacts to upgrade the network (Sestino et al., 2020). To perform such actions, the companies must have a strong grip on the provenance and data consumption that is mostly used in such cases, and precisely on point. To meet the business demands the organizations must hire a BI consultant that can guide in the establishment of all data matrices.
Never pigeonhole your BI Data consultant
Power BI data consultants are most often sequestered within an organization because the data consultants and business leaders don’t know each other. Data analytics projects cannot survive if they perform their duties separately from the rest of the business. The consultant who successfully copes with this challenge has to do it mostly in two ways. The first one is to break boundaries between the business and data analytics (Božič et al., 2019). One leading organization can rotate staff from the center of excellence and into the line roles where they can measure the scale of concepts.
Then, they have to return to the center. A global organization can design new roles in different functional areas and business lines where they can augment analytical sophistication. These roles act as a dotted line between the center of excellence relationship. Companies from the leading side use one other tactic where they drag the data analytics and data consultants towards the business or pull the business towards data consultants by insisting the employees discuss quantitative topics (Božič et al., 2019). But the leaders of data culture organizations can not stay away from the data language.
Try to Fix basic data access issues
We mostly face complaints that the individuals in different departments within the business are facing issues and trying the access the most basic part of the data. This situation exists despite having a lot of efforts to democratize the data access within the organizations. Sometimes the data analyst did not adopt proper approaches for data-driven culture. Top organizations use simple and basic strategies to break traditional concepts (Paais et al., 2019). To reorganize the data, the data analyst grants universal access to just key measures at a time. For example, a global bank is trying to introduce better services for loan refinancing needs and construct a standard data layer for its marketing department by focusing only on some relevant measures (Paais et al., 2019). So, in this matter, the key terms for data pertaining are property information, balances, data for customer characterization, marketing channel to originate the loans and a canny choice for the data to make it accessible using the metrics which are suited to the organization agenda. Moreover, the other factors associated with this data source can also encourage to use of this approach.
Quantify data uncertainties
Everyone accepts the concept that it's impossible to achieve absolute certainty. However, most of the managers continue to ask their team members to answer the questions without the corresponding confidence measure. Demanding a team to have quantitative and explicit certainty levels has three major and powerful effects. First, it can force the decision-makers to directly consider the potential sources of uncertainty. Is the required data reliable? They have a few examples of the reliable model.
How some factors can be incorporated when no data exists for them such as the competitive emerging dynamics. For example, the one retailer comes to an analysis that the downgrading of the redemption rates from direct marketing models can be caused by enhancing the stale address data. Updating the data and keeping the process of data up to date can fix a lot of problems (Božič et al., 2019).
Second, the power BI data analysts have a deep understanding of their models when they have to evaluate uncertainty. For example, a UK core risk model had failed to adjust market trends with time.
So, there is a need to build an early warning system into account otherwise they have to face some consequences.
Third, and final, they should have to emphasize understanding the uncertainty that the organizations required to run experiments. In some cases, test and run mean business success. So, the team of quantitative analysts has to pair up with the category managers to conduct efficient and controlled trials on their ideas before making any widespread changes.
Make the proof of concepts
In data analytics, a better approach is to the user the proof of concepts when the core concept part is visible in production. One good method is to try to develop something that is considered industrial-grade but simple and later can be used up to the level of sophistication. For example, one client wanted to implement a basic risk model at a large scale and distributed computing systems. Data-driven organizations started to implement a basic method that works from end-to-end sources.
During this process, a small data set flows from the source system and uses a simple model and is delivered to end-users. Once it was in a right place, the whole system is covered, and the organization can enhance each component individually with greater data volume, data models and better runtime performance (Paais et al., 2019).
Should offer specialized training
Many companies invest a large amount of money in training efforts for their employees to rapidly implement their strategies about what they have learned if they have not used the required skillset inappropriate ways. So, using a basic skill such as coding should be a part of fundamental training. It will be more effective if the employees are trained using the specialized analytical tools and concepts just before the use of analytical skills is required for the proof of concept. For example, one retailer waited before the first market trial and was trained about the supporting analytics in the finer stage of the experimental designs. The knowledge can be shared across the team and become a part of the team’s vernacular (Božič et al., 2019).
Use analytics to help employees
It is considered easy to forget the data fluency role in the marketing employees. But empowering the employees for data wrangling can do this and can enable them to follow the suggestions in a memorably titled book such as the “Automate the Boring Stuff with Python”. If the idea to learn new things is presented in a better manner, some employees will be excited even to revamp or preserve their work (Sestino et al., 2020). However, if the immediate goal is to take benefits directly by saving valuable time, avoiding rework, or extracting the most desired information, then a chore will become a choice. Some years ago, the analytics team could only access data through their IT department, in current times it is prudent to allow the BI team some cloud computing resource, so that they can experiment with the large dataset using new models without waiting for the IT department to take action according to their requirements (Sestino et al., 2020). This approach has proved to be useful and many IT departments have remade the organizational technical infrastructure, to enable easier access to data and analytics. When the time will come to figure out the requirements of the platform for advanced data analytics, the team has to do more than just describe answers. They have to demonstrate the working solutions.
Always try to trade with flexibility and consistency
Many companies depend on different data tribes. Each organization uses its preferred data sources of bespoke metrics, information and most beneficial programing language. If proper measurements are not taken then it will be a disaster for an organization (Heavin et al., 2018). Companies waste a large number of sources to try to reconcile the different versions of metrics that can be universal. Inconsistencies in their models to work can also take a long time. Moreover, if the coding standards and time vary across a business, every move of analytical talent can entail retaining and make it hard to circulate for them. It also becomes difficult for them to share ideas internally if they required translation every time (Heavin et al., 2018). For this purpose, companies have to select the programming language and canonical metrics. One of the leading global banks adopted this approach by insisting the new hires in bank investments and asset management are proficient with data analysis using python.
Try to explain analytical choices
To solve the analytical problems, there is rarely a single and correct approach. Data analytics consultants adopt different approaches with tradeoffs. So, it is considered a good idea to discuss with the team how a problem can be addressed, what are the possible alternatives can be considered, what are the tradeoffs they can understand and why they have selected one particular solution over others (Tabesh et al., 2019). Doing this can give the organization a deeper understanding of the approach taken and often demonstrates the consideration of alternative options to retain assumptions.
Conclusion
Data consultants and analytics is more than the methods, AI, machine learning, and relevant tools. To make your organization adopt new emerging technologies, organizations have to make necessary amendments to the existing ecosystems to enable a data-driven culture. Defining the teams, aligned objectives, mindsets and approaches toward data analytics will open new doors for your business.

Leon Gordon, Executive Contributor Brainz Magazine
Leon Gordon, is a leader in data analytics. A current Microsoft Data Platform MVP based in the UK and a Partner at Pomerol Partners (pomerolpartners.com). During the last decade, he has helped organizations improve their business performance, use data more intelligently, and understand the implications of new technologies such as artificial intelligence and big data.
Leon is a Thought Leader at the Forbes Technical Council, an Executive Contributor to Brainz Magazine, a Thought Leader in Data Science for the Global AI Hub, chair for the Microsoft Power BI – UK community group and the DataDNA data visualization community as well as an international speaker and advisor.
References
Tabesh, P., Mousavidin, E., & Hasani, S. (2019). Implementing big data strategies: A managerial perspective. Business Horizons, 62(3), 347-358.
Heavin, C., & Power, D. J. (2018). Challenges for digital transformation–towards a conceptual decision support guide for managers. Journal of Decision Systems, 27(sup1), 38-45.
Sestino, A., Prete, M. I., Piper, L., & Guido, G. (2020). Internet of Things and Big Data as enablers for business digitalization strategies. Technovation, 98, 102173.
Božič, K., & Dimovski, V. (2019). Business intelligence and analytics for value creation: The role of absorptive capacity. International journal of information management, 46, 93-103.
Paais, M., & Pattiruhu, J. R. (2020). Effect of motivation, leadership, and organizational culture on satisfaction and employee performance. The Journal of Asian Finance, Economics, and Business, 7(8), 577-588.